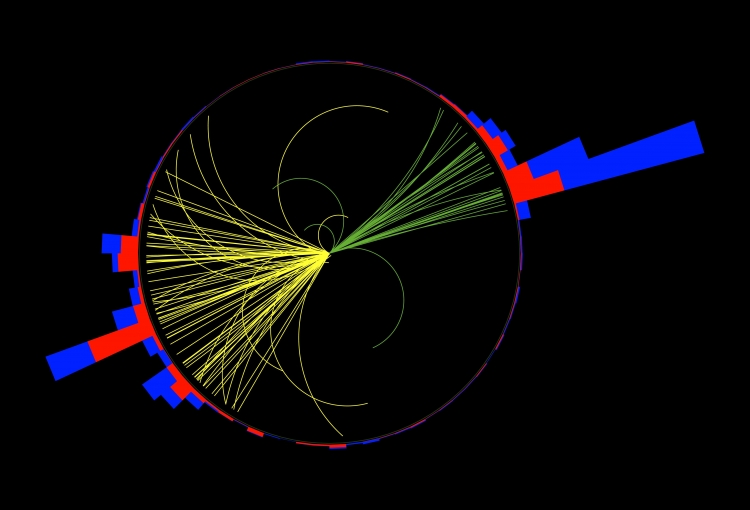
New algorithms from the Compact Muon Solenoid experiment use the ideas used in mobile phone facial recognition to better understand the collisions at the Large Hadron Collider.
One of the most exciting challenges at the Large Hadron Collider is the identification of our events. We must reconstruct particles that live for a tiny fraction of a second by looking at the detection of their decay products. These decay products often produce sprays or “jets” of particles, as shown in Figure 1. If the original particle has a high momentum, the jets originating from its decay products will merge. In order to perform our analyses, it is necessary to identify what kind of particle (Higgs bosons, W/Z bosons, quarks, gluons, etc) created the jets in our event.
Figure 1: Particle “jets” are created from proton-proton collisions at the LHC. In order to reconstruct a collision, it is necessary to determine what kind of particles created the jets. In this case, two top quarks decay. Each top quark decays into three separate quarks that merge together into one large jet.
This is very similar to facial recognition. Perhaps your tablet can recognize your face in order to unlock itself, which works by using advanced computer programs implementing “machine learning” to extract features from your face. When you first purchase your tablet, you activate a special “training” mode to teach it what your face looks like. This works by extracting features from your face automatically and then passing them to decision-making algorithms to determine where they come from.
We can use the same machine learning techniques to identify the origins of jets. For instance, we can look at the energy and direction measurements of the particles within a jet and project it onto two dimensions so it looks like a regular image. The same algorithms that can tell the difference between two different faces can also tell the difference between different types of jets. One particularly useful example is how many “hot spots” exist within the jet. It turns out that heavier particles (like Higgs bosons or top quarks) will have multiple “hot spots” (like in Figure 2), whereas lighter particles (like up quarks or gluons) tend to have only one (like in Figure 3). In Figures 2 and 3, we overlay many events to show features that are difficult to see in a single event.
![]() |
![]() |
Figure 2: We can distinguish different kinds of particles by the images of their resulting jets. On the left is the overlay of many jets from lighter quarks and gluons, resulting in one “hot spot” of activity, and on the right is an overlay of many jets from massive top quarks, resulting in three “hot spots” of activity. The same techniques that are used for facial recognition can be used to tell the difference between different types of jets.
These new and innovative techniques show great promise in increasing our capability to identify the origins of different jets. For instance, the probability to correctly identify jets from massive top quark decays can be increased by around 33%. These advancements make our analyses more sensitive and improve the capabilities of discovering new physical interactions.
In the upcoming Run 3 of the Large Hadron Collider that starts in 2021, and beyond that in the further future such as High-Luminosity LHC, these techniques will be increasingly important in improving sensitivity to searches for new physics that interacts with Higgs/W/Z bosons and top quarks. In addition, they will also play a large role in further studies of the detailed behaviour of the Higgs boson and its decay to quarks.
Read more about these results in the CMS Physics Analysis Summaries:
- CMS Paper "Identification of heavy, energetic, hadronically decaying particles using machine-learning techniques"
- This article was originally written for CMS Physics Analysis Summary JME-18-002: Machine learning-based identification of highly Lorentz-boosted hadronically decaying particles at the CMS experiment
- All CMS physics briefings
- All CMS preliminary results
- All CMS results
- Log in to post comments