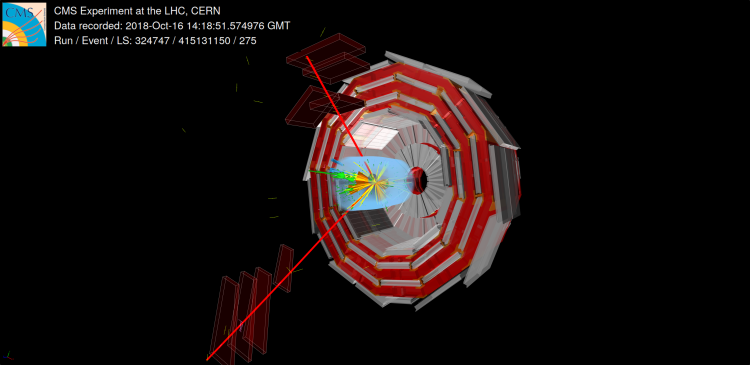
The CMS experiment at the LHC is set to explore the fundamental nature of our universe. It allows scientists to study particles, their interactions, and the forces that shape our world. Through these studies, a deeper understanding of the building blocks of matter and the secrets of the cosmos is reached.
CMS stands for Compact Muon Solenoid, having been especially designed to detect muons. A muon is a type of elementary particle, similar to an electron but 200 times heavier. Muons have special properties that make them useful for scientific research. For example, muons can travel through several layers of detector material, allowing CMS to detect them in the most external part of the detector, about 7 m away from the place where protons collide. The trajectory of a muon in CMS is shown in Fig. 1.
Figure 1. Scheme of a muon passing through different layers of the CMS detector and put its signature in muon stations.
As a result, muons provide a clear signal and therefore play a crucial role in the CMS experiment. They are first observed in the innermost part of the detector, the tracker, where their trajectories can be measured with great precision. They are then also detected in the outermost part of the detector, the muon chambers; the majority of particles to be detected in these chambers are muons, making it relatively easy to identify a particle as a muon and improve the precision of the trajectory even further. By combining the information in both parts of the detector, muons can be identified with high quality.
When protons collide at high energy in the LHC, many particles are created. Most of them are not stable and decay into other particles, such as muons. There are many studies where the presence of a muon suggests that an interesting event has been produced at the LHC. For example, it might indicate the presence of a Higgs boson. Besides, not all muons are equal, each having different properties depending on the particle they come from. In addition, sometimes other particles can be wrongly identified as muons, compromising the recognition of real and good muons. Therefore, improvements in our ability to correctly identify muons will greatly impact the quality of the CMS results. A couple of machine learning (ML) methods have been used by CMS to overcome the challenges of muon identification. This is one of many use cases where ML surpasses, in performance, the methods traditionally used in experimental particle physics.
The first technique is known as ‘MVA ID’ (for “MultiVariate Analysis IDentification”) and is designed to discriminate muons from other types of particles, like light mass hadrons, which can reach the muon chambers and be incorrectly identified as a muon. The model is trained using the tracks left by both real muons and light hadrons in the detector, so it can learn the differences. Hence the model, when provided with a muon candidate, can decide if it is likely a real muon or not. This model provides a new muon identification criteria that has been proven to select muons more efficiently than the standard identification used so far. This can be seen in Fig. 2, which shows the probability of correctly selecting a real muon (true positive rate) versus the probability of misidentification (false positive rate). One can see that, for a given misidentification rate, the MVA ID provides a higher probability than the traditional (cut-based) methods to correctly select muons. Besides, this new technique is more flexible, as it gives us many options: one can move on the curve and find the sweet spot on the quality requirements that is suitable for a specific physics analysis.
Figure 2. Performance of the Muon MVA ID (black line and stars) in comparison with other existing muon selectors (filled and open circles).
At the LHC, proton collisions are made by throwing bunches of billions of protons to each other. This maximizes the chance that at least two protons meet each other and collide. At the same time, this may result in multiple proton-pair collisions being produced at once, which complicates the task of understanding which collision generated what in the CMS detector. This was a challenge already for the Higgs discovery (with a handful of collisions happening at once), it became an even bigger challenge during data taking in 2015-2018 (up to 40 collisions at once, on average) and it is even worse in the ongoing data taking period (60 collisions on average expected for 2024). In this crowded environment the task of identifying muons will be even more difficult. This machine learning model is able to distinguish real muons very efficiently, even in those challenging conditions.
The second machine learning model is called ‘Prompt-muon MVA’ and focuses on selecting a very specific type of muons, denoted as “prompt muons”, that come from the decay of the W, Z, or Higgs bosons, and of tau leptons. This type of muon has an important feature: it is most of the time isolated, i.e., not surrounded by other particles. This is completely different from what happens, for instance, with muons from a b-quark decay, that are produced with a stream of other, close-by, particles. The Prompt-muon MVA is trained to distinguish muons from Higgs/W/Z bosons and taus from muons coming from b-quark decays. In order to do so, the ML model uses the information that the detector has collected in the surroundings of the muon candidate. A comparison of the performance of the Prompt-muon MVA with other isolation criteria can be seen in Fig. 3, where the MVA method pushes the non-prompt rate to very low values. This ML model has already been successfully used in CMS to analyze data from the years 2016 to 2018.
It was a key to the first observation of the simultaneous production of a Higgs boson and two top quarks, with top quarks and the Higgs boson eventually decaying to leptons. In addition, it contributed to the recent observation of “four-tops” in CMS, where four of the most massive known particle, the top quark, are produced simultaneously. Given the low frequency of these processes, those measurements were very challenging, but the ability of this ML model to identify muons coming from signal processes pushed significantly the sensitivity of the study, and allowed the CMS collaboration to claim those observations.
Figure 3. Performance of the Prompt-muon MVA (black line and black circle) in comparison with other identification+isolation criteria (green and blue lines).
In summary, the aforementioned ML methods further improve the muon selection in CMS. This is crucial to improve the accuracy of data analyses, enabling better signal selection and background rejection. Ultimately, this will lead to more precise measurements of how particles interact and may even reveal the existence of new particles not predicted by our current theory, the standard model of particle physics.
Read more about these results:
-
CMS Physics Analysis Summary "Identification of prompt and isolated muons using multivariate techniques at the CMS experiment in proton-proton collisions at 13 TeV"
-
@CMSExperiment on social media: LinkedIn - facebook - twitter - instagram
- Do you like these briefings and want to get an email notification when there is a new one? Subscribe here