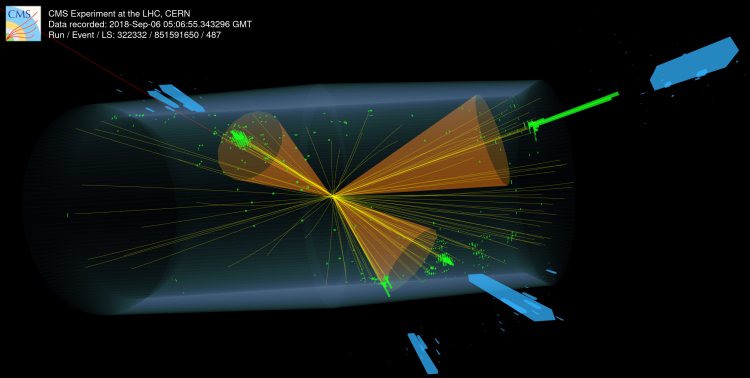
One of the events determined by the AI algorithm to be highly anomalous and therefore potentially coming from a new particle. The central jet shows particles clustered around two different cores within the cone, a configuration distinct from a typical jet in which particles cluster towards the center.
One of the main goals of the CMS experiment is to look for signs of new particles, which would help explain many of the outstanding mysteries of the cosmos. When the high-energy protons from the LHC collide inside the CMS detector, their energy can briefly be converted into yet-undiscovered particles, which typically quickly decay back to familiar matter. Since such particles will be produced extremely rarely, advanced techniques are needed to search for them. A great deal of effort is spent by CMS analyzers in designing sophisticated statistical analyses to search for the signs of different hypothetical particles. However, because the data is so vast and complex, and the new particles so rare, these analyses usually must be designed to look for one specific type of new particle at a time. But what if we aren’t seeing new particles because we haven’t thought of the right ones to look for? Could an AI-led search help us find new particles that the many human-led efforts have missed?
This latest search from the CMS Collaboration tried such an approach. They selected collisions with an extremely common signature, two collimated sprays of particles called ‘jets’, and then let AI algorithms take the wheel. The AI algorithms picked the collisions whose jets looked the most ‘anomalous’ as compared to regular ones and then an automated statistical analysis was performed to see if these anomalous jets were actually evidence of new particles.
While AI has been used many times by CMS before, previously it was always trained to identify the signatures of a specific particle chosen by the analyzers. Training this type of AI algorithm uses simulated collisions producing the hypothetical particle as labeled examples for the AI algorithm to learn from. Instead, “this analysis is the first time CMS has trained an AI solely on the collision data itself, without any guidance from the analyzers on what new particle to look for”, said Oz Amram, from the CMS analysis team.
Training an AI algorithm without labeled examples is a tricky endeavor. The analyzers employed multiple algorithms based on three different strategies to ensure sensitivity to as many different signatures as possible. One strategy was to train the AI algorithm on a data sample of regular jets and then ask the AI to pick out the jets that looked the most out of the ordinary as compared to those. Another strategy tasked the AI algorithm with distinguishing between two groups of collisions, only one of which may contain a small fraction of new particles. The algorithm would then learn how to identify the unique signature of the new particles as compared to regular jets. The final approach created a ‘prior’ of what new signals could look like by using simulated examples and then asked the AI to find collisions that looked distinct from regular jets and similar to the signal prior. Each was found to have varying sensitivities to different types of new particles and no algorithm was found to be optimal for all potential signals.
Figure 1: A summary of the different AI algorithms used to search for anomalous jets.
If there had been a new particle of certain mass producing anomalous jets, it would have shown up as a ‘bump’, or cluster of anomalous events at a certain energy, which indicated their common origin. The statistical analysis used to look for evidence of such a bump had to be automated because the analyzers did not know ahead of time what kinds of events would be selected as anomalous by the AI algorithms. “We did extensive testing in simulation and signal-free parts of the data to ensure that the AI algorithms would not artificially create bumps which could be mistaken for a signal”, said Sam Bright-Thonney, another member of the CMS team. Studies also showed the AI algorithms were able to successfully identify the signatures of new particles if they were present in the data. They were found to perform better than several traditional approaches and therefore were capable of facilitating discoveries that may otherwise have been missed.
Excitement was high when the AI algorithms were finally unleashed on the data, but “unfortunately none of them found any significant evidence for new particles”, said Manuel Sommerhalder, another CMS physicist.
Figure 2: Mass distribution of the events that one AI algorithm selected as most anomalous. A new particle would have appeared as a ‘bump’, or excess of events at a certain mass value, on top of a smooth distribution of background events. Example bumps from two different signals are shown as blue and purple dashed lines. No such bump was observed.
However, the results did allow limits to be placed on the rate of producing several different types of particles that would have produced anomalous jets. It was also shown that the AI-led algorithms significantly enhanced the discovery sensitivity to a wide range of particle signatures as compared to traditional techniques.
Figure 3: A comparison of the rate of anomalous collisions (cross section) that would be needed to discover four different hypothetical new particles (bottom labels). Traditional strategies that analyzed all events with two jets (black) or looked for jets with particular characteristics (brown and tan) are compared to the multiple AI-led algorithms shown in the various colors. The bottom panel shows the improvement of the AI-led algorithms as compared to the traditional ones. It shows that they are able to make discoveries with much fewer signal events (up to a factor of 7), increasing the sensitivity to rare new particles of different types.
This search was the first from CMS to use these advanced AI techniques, but it won’t be the last. “We already have ideas about how to further improve the algorithms and apply them to different parts of the data to search for several kinds of particles,” added Amram. Rather than putting them out of a job, letting AI take the lead is giving physicists new ways to analyze their data and could soon enable new discoveries!
Read more about these results:
-
CMS Physics Analysis Summary (EXO-22-026): "Model-agnostic search for dijet resonances with anomalous jet substructure in proton-proton collisions at 13 TeV "
-
Display of collision events: CERN CDS
-
@CMSExperiment on social media: LinkedIn - facebook - twitter - instagram
- Do you like these briefings and want to get an email notification when there is a new one? Subscribe here